POV
Transforming the Pharma Commercial Function with Generative AI
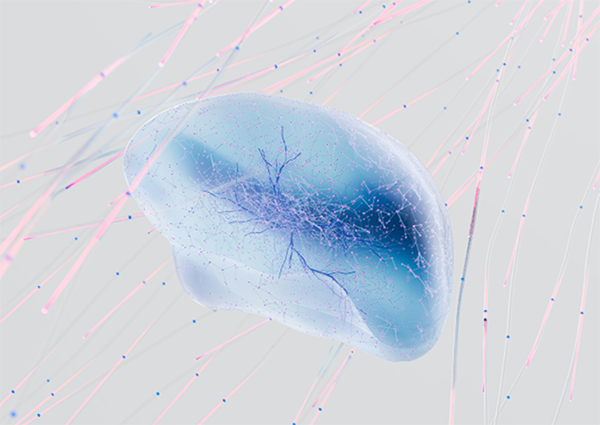
Since ChatGPT and similar AI technologies went mainstream, leaders across various industries have been exploring their impact on current and future business plans. The life sciences sector is particularly active in exploring the potential benefits that generative AI offers.
This technology adds another layer of complexity for pharma leaders and omnichannel marketers. Life science brands are already deeply involved in digital transformation, adopting new communication methods to engage with healthcare professionals (HCPs). Plus, they’re under constant pressure to optimize their spending while still trying to meet and exceed their revenue targets.
In short, all companies today are struggling to understand how generative AI (a.k.a. Large Language Models, or LLMs) fits into their organizations and how to use it in the most effective and efficient ways, with the least amount of investment and risk.
Commercial teams are developing their own “use cases” for deploying LLMs. These initial applications target specific tasks to help reduce administrative burdens and optimize people’s time and resources. Examples of use cases include:
- Administrative Optimization: Early adopters are likely to use LLMs for administrative tasks where deep domain-specific knowledge isn't crucial. For example:
- Conversational Search:Teams can use LLMs to carry out complex searches, improving the efficiency and accuracy of retrieving information, such as parsing through open-text responses in surveys.
- AI Assistants for Research and Ideation: LLMs can help generate research summaries, cutting down the time teams need to gather and analyze information, while speeding up tasks like competitive claims research.
- Automated Content and Asset Creation: A major strength of LLMs is their ability to create text-based and, increasingly, visual content.
- Accelerated Content Ideation and Delivery:LLMs can produce several content options faster than humans. For instance, LLMs can automate the generation of different banner ad versions, streamlining the design process and incorporating elements like brand tone and voice as the technology evolves.
- Automated/Assisted Data Analysis: : LLMs' ability to process and interpret data can be used to automate routine data analysis tasks. One example? Sending data directly to team inboxes, eliminating manual work.
- Domain-Specific Training: While mainstream LLMs are trained on broad and diverse datasets, they often lack training on industry-specific data. This can hinder their ability to deliver accurate and contextually relevant responses in specialized fields.
- Relevant Timeframes: LLMs process only the data they have been exposed to. Frequently, this data may be outdated, which can affect the accuracy of the model's responses.
- Accuracy Issues: Although LLMs can produce responses that appear logical, they can be significantly off the mark, especially when evaluated by field experts. This inaccuracy often arises from the model's limited realistic, contextual grasp of specialized subjects.
- Response Ambiguity: LLMs operate based on "prompts" or questions posed by users. This mechanism is more problematic than it might seem, as minor variations in how questions are phrased can lead to different, and sometimes unclear, responses. This variability is particularly challenging in highly regulated sectors where clear and precise communication is essential.
- Proprietary Data Requirements: The life sciences industry relies on confidential, non-public data. Using LLMs in specialized areas like drug discovery, precision medicine, and clinical trial optimization requires training the models on these specific, internal datasets. This necessity adds layers of complexity, both in terms of logistics and regulatory compliance.
Which LLM implementations will drive the most impact? Where can brands automate for a consistent output performance? Which decisions to make and how to allocate resources depends on each organization, through testing and learning.
If your enterprise is considering launching its own version of an LLM, consider the following high-level steps:
- Foundational Model Use: Start with an existing AI model as the base for your generative AI application.
- Customized Training: Adapt the model to meet your specific requirements, including using your proprietary content and aligning with your brand’s tone and voice.
- Creation: Build a comprehensive prompt library and thoroughly test it to ensure it interacts correctly with the AI model.
- Application: Use the prompt library as intended, whether for direct interactions with the tool or to automate the delivery of responses to your inbox.
- AI Engineers: Tasked with developing, implementing, and managing the AI model.
- Data Modelers: Focused on organizing and maintaining the data needed for the AI model’s training and functionality.
- Functional Subject Matter Experts (SMEs): Provides crucial industry-specific and brand-specific insights to guide the AI model's training and usage.
- DevOps Team: Critical in deploying and maintaining AI applications, ensuring they integrate smoothly with existing systems and remain reliable and operational.
When deciding whether to build or buy a Generative AI capability, enterprises must carefully evaluate several factors:
- Build: Building offers full control and customization, ensuring data security and IP ownership. For faster time-to-market, enterprises can use OpenAI embeddings to represent their proprietary data in a format compatible with pre-trained language models.
- Buy: Buying provides rapid deployment and immediate access to AI capabilities, leveraging vendor expertise and support. For instance, consider content tools such as Jasper.AI and Writer.AI, and domain-specific LLMs such as Ferma.AI and Huma.AI
While LLMs hold promise for streamlining administrative tasks, from research assistance to content creation and data interpretation, life science brands need to recognize their limitations. Response ambiguity, a lack of domain-specific training, and the need for training models on specific, internal datasets are just a few of the challenges brands will face when implementing generative AI.
In short, while LLMs can help automate many routine tasks and provide insights, they cannot replace the critical and strategic thinking that brands require to stay successful.
Ready to elevate your brand with a cutting-edge omnichannel strategy?Connect with Asentech today, and discover how we can guide you toward omnichannel excellence for a successful launch.