POV
Gen AI Unleashed: A Spectrum of Use Cases in Pharma Marketing and Commercial Operations.
Opportunities, Challenges, and Path Forward
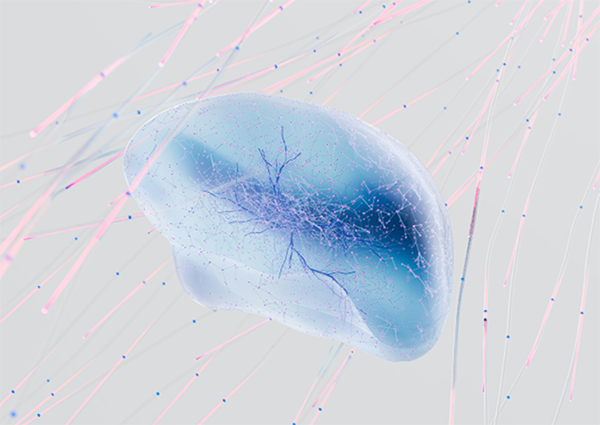
Since ChatGPT and its many competitors have become mainstream, industry leaders have been diligently seeking to understand how generative AI will affect their present and future business objectives. Life Sciences is certainly participating in this dynamic and clearly has significant opportunities ahead.
Todayâs situation is very complex for most Commercial life sciences teams. They have been engaged in an intensive process of digital transformation, to adapt and leverage new communication channels, and new HCP engagement and media consumption patterns. Plus, there is constant SG&A pressure to optimize their spending while simultaneously meeting and exceeding their revenue goals.
The arrival of generative AI adds another layer of complexity and compels these leaders to further push the boundaries of transformation. They are now faced with the task of understanding the implications of generative AI within their functional scope â and how to gain the most transformational leverage with the lowest investment and risk.
Generative AI, also referred to as Large Language Models (LLMs) will be groundbreaking in many aspects, however they do present certain limitations that are particularly important in specialized fields like Life Sciences. These early considerations fall into several categories:
- Domain-Specific Training: Mainstream LLMs have been trained on expansive, diverse datasets, but usually not on industry or domain-specific data. This can limit their ability to produce accurate and contextually appropriate responses in specialized fields.
- Relevant Timeframes: LLMs can only process data or content to which they have been exposed. Often that input information is dated or may be cut off at a certain point in time, which can skew the modelâs responses.
- Accuracy: Although LLMs can generate responses that seem logical, these responses can often be significantly inaccurate, particularly when assessed by experts in each field. This stems from the model's lack of realistic, contextual understanding of specialized subject matters.
- Response Ambiguity: LLMs require âpromptsâ which are questions the user wants answered. This is more problematic than many leaders realize because slight variations in prompts, even with similar intent, can lead to differing and potentially ambiguous responses. This inconsistency can pose challenges, especially in highly regulated industries where precision and unambiguous communication is crucial.
- Proprietary Data Requirements: Industries like Life Sciences rely on proprietary, non-public datasets. To apply LLMs in specialized areas such as drug discovery, precision medicine, and clinical trial optimization will require training the model on these domain-specific, internal datasets, which introduces more complexity, both logistically and from a regulatory perspective.
These limitations underline the challenges that leaders will face when aiming to leverage the full potential of LLMs. They also emphasize the need for further refinements in these models to enhance their applicability in specialized fields.
Commercial teams are developing their own âuse casesâ for the first LLM deployments. These early implementations are focusing on specific tasks that can help reduce administrative burdens and make better use of peopleâs time and resources. At this point Clinical use cases generally will fall outside of Commercial, due to the nature of scientific work and regulatory compliance. Potential implications and use cases are, for example:
Administrative Optimization
Early adopters will likely focus on administrative applications to streamline tasks that do not require deep domain-specific knowledge.
- Conversational Search: Teams can leverage LLMs to perform complex searches, potentially enhancing the efficiency and efficacy of information retrieval, say within verbatim open-text survey responses.
- AI Assistants for Research and Ideation: LLMs can be utilized to generate research summaries, significantly reducing the time required by teams to collate and analyze information. As an example, competitive claims research studies could be accelerated.
Automated Content and Asset Creation
One of the key strengths of LLMs is their ability to generate text-based content, and increasingly, visual content.
- Accelerated Content Ideation and Delivery: Teams can utilize specialized LLMs to enhance the content ideation process to produce variations and choices more quickly. As an example, LLMs can be used to automate the creation of multiple versions of banner ads, saving time and resources in the design process. Eventually this will include sophisticated nuances like brand tones and voices.
Automated/Assisted Data Analysis
The ability of LLMs to ingest and interpret data can be exploited to automate routine data analysis tasks.
- Analytics Requests Teams can automate routine but sophisticated analytics requests using LLMs, enabling important data to be delivered directly to their inboxes without the need for human analyst involvement.
These potential use cases demonstrate the adaptability of LLMs and their potential value in enhancing productivity and efficiency within commercial teams. While these applications are focused on administrative and time savings for now, more complex tasks are soon to be seen.
Implementing LLMs for Commercial teams will require a variety of strategic decisions and the allocation of specific resources. Much of the decision making will revolve around testing and learning where there is most impact along with consistent output performance.
Model Selection and Training
- Foundational Model Use: Employ a pre-existing AI model as the base for your generative AI application.
- Customized Training: Tailor the model to your specific needs, including proprietary content types, and brand's tone and voice.
Prompt Library
- Creation: Develop and extensively evaluate a comprehensive prompt library to facilitate interaction with the AI model.
- Application: Utilize the prompt library for the intended purpose, whether it's direct interaction with the tool or scheduling delivery of responses to your inbox.
Team Training and Potential New Roles
- AI Engineers: Responsible for developing, implementing, and managing the AI model.
- Data Modelers: In charge of structuring and maintaining the data required for AI model training and operation.
- Functional Subject Matter Experts (SMEs): Will provide essential industry and brand-specific knowledge to inform the AI model's training and application.
- DevOps Team: Will play a critical role in deploying and maintaining AI applications, ensuring their seamless integration with existing systems and overseeing their continuous operation and reliability.
- Internal Development: Some aspects of the AI application may need to be developed in-house due to the need for training on proprietary datasets.
- External Tools: A wide variety of tools are available that can be integrated into your ecosystem to meet your objectives. (For context, there are content tools like Jasper.AI and Writer.AI; LLMs like OpenAI embeddings; and domain specific LLMs like Ferma.AI and Huma.AI, among others.)
By carefully considering these points and allocating resources appropriately, Life Sciences commercial teams can effectively deploy generative AI applications to enhance their operations and achieve their goals.
In summary, the advent of LLMs such as ChatGPT brings both opportunities and challenges for the Life Sciences industry. The inherent limitations of LLMs, including their lack of domain-specific training and the potential for ambiguous responses, must be recognized. However, they hold promise for streamlining administrative tasks, from research assistance to content creation and data interpretation.
Deploying generative AI applications demands strategic decisions and resource allocation, from utilizing foundational models to customized training, prompt library development, and training and developing new skills and roles on the team. The decision to build in-house or utilize external AI tools must also be carefully evaluated against speed to value.
In conclusion, despite the challenges, with careful planning and strategy, Life Sciences commercial teams can successfully harness the power of generative AI, positioning themselves at the forefront, and continue growing into this technological revolution.